Neurobiology
How machine intelligence helps in translating the neural code
What happens when visually responsive neurons in the primate brain are allowed to interact with artificial neural networks that generate images?
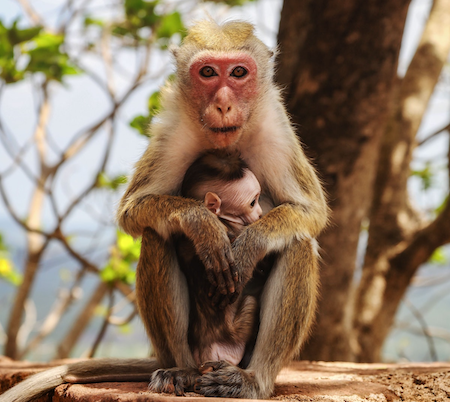
When we look at the world before us, we experience a unified scene of shapes and colors. But individual neurons in our brain do not. They are microscopic automata that can only emit electrical impulses when specific shape patterns appear in their little territory of visual space. Billions of these neurons work together to give rise to visual recognition, and yet they are still outnumbered by the practical infinity of images impinging on the retina over time. That means that the brain must be efficient about allocating its neurons - it cannot assign a neuron to detect every pattern in the world. Neurons should be selective about which shape patterns to learn to recognize in scenes.
The foundation of visual neuroscience rests on understanding the selectivity of individual neurons. So how do we discover what any given cell signals about the world? Most of us use a robust experimental design developed by David Hubel and Torsten Wiesel in the 1950s, where an animal faces a screen that displays pre-selected pictures. Meanwhile, microelectrodes placed close to neurons in the brain report the presence of action potentials ("spikes"). But experimental sessions are time-limited, and therefore investigators must have a good idea of what pictures to show the neurons in the first place. Lines, angles, pictures of faces, animals, places...miss the right image, miss the correct conclusion. The problem is that there is an astronomical number of pictures worth testing.
What if instead of trying to guess what a neuron encodes, we just let it "tell" us by guiding the development of images based on its own responses? This concept was the core idea behind our project. Our co-authors Will Xiao and Gabriel Kreiman had been working with new artificial neural networks that can generate images from scratch. These artificial neural networks are called generative adversarial networks (GANs). They found that these image generators could be guided into creating images that made computational model "neurons" respond more strongly than to any other picture.
As part of the Margaret Livingstone laboratory, we set out to discover if this could also work in biological neurons. We used a GAN pre-trained by Jeff Clune's team at the University of Wyoming, which functioned by taking in a compact list of numbers (an input code) and outputting an image. Each experiment began as we inputted 40 codes into the GAN, which created images of formless textures. The textures were presented to a monkey keeping a steady gaze on the screen, which elicited responses from neurons in the monkey's cortex. After 2-3 minutes, these responses were used by an algorithm to identify which codes were better than others - codes leading to images that elicited more spikes were worth keeping and modifying slightly for the next generation of images (if this reminds you of evolution, you are right: this is a so-called genetic algorithm). Over tens of minutes, we found that the neuron led the GAN into creating formed images. First selecting a dark spot, then another aligned horizontally, then a convex line, surrounding them both from above. The result was something like a face, which made sense to us because that particular cell also responded strongly to pictures of actual faces. Yet the neuron responded to this synthetic image more so than to any face pictures.
We replicated this process across neurons and individual monkeys. The neuron-driven synthetic images are a fascinating mix of the familiar and the bizarre. Many contain patterns we can easily recognize in monkeys - face-like patterns surrounded by the tan-reddish brown textures like monkey fur - but many contain patterns that we cannot readily refer to any given object category. We can interpret these synthetic patterns by showing them to biological and artificial neurons and comparing how these neurons rank the synthetic patterns relative to photographs of real-world objects. Both biological and artificial neurons confirm that many of these synthetic patterns are present in pictures of real objects, especially monkeys and other animals, consistent with our understanding of monkeys as highly social animals.
Machine learning has become a boon in solving the 70-year-old problem of visual selectivity in the primate brain. Currently, we are working to identify the full diversity of patterns represented in the macaque brain, extending from Hubel and Wiesel's primary visual cortex to the end of the visual recognition pathway at the inferotemporal cortex. Finding this full set of patterns - what some scientists have called the visual alphabet - will hopefully lead to deep network models that better resemble the primate brain.
Original Article:
Ponce C, Xiao W, Schade P, Hartmann T, Kreiman G, Livingstone M. Evolving Images for Visual Neurons Using a Deep Generative Network Reveals Coding Principles and Neuronal Preferences. Cell. 2019;177(4):999-1009.e10.Edited by:
Massimo Caine , Founder and Director
We thought you might like
Hacking the tryptophan metabolic process to reduce neurodegeneration
Apr 25, 2017 in Health & Physiology | 3 min read by Carlo BredaCould we reverse memory loss in Alzheimer’s patients? Mice answer yes!
Mar 16, 2017 in Health & Physiology | 2.5 min read by Aude Marzo , Faye McLeod , Patricia SalinasThe power of our adaptive immunity against Alzheimer’s Disease
May 10, 2017 in Health & Physiology | 3 min read by Daniele GuidoMore from Neurobiology
New, smaller-than-ever devices to help us understand how our brain works from the inside
Nov 8, 2024 in Neurobiology | 4 min read by Filippo DonatiCan we use a magnet to see brain inflammation?
Sep 25, 2023 in Neurobiology | 4 min read by Raquel Garcia-Hernandez , Santiago Canals , Silvia de SantisSurprising Behavior Changes in Genetically Modified Syrian Hamsters
Aug 30, 2023 in Neurobiology | 4 min read by Susan Lee , Kim Huhman , Jack TaylorTo achieve goals, we definitively need our neurons
Mar 10, 2023 in Neurobiology | 3.5 min read by Julien CourtinThe Impact of SARS-CoV-2 on the Brain: It Is All in Your Head
Feb 15, 2023 in Neurobiology | 3.5 min read by Meredith G. Mayer , Tracy FischerEditor's picks
Trending now
Popular topics