Maths, Physics & Chemistry
How robots can learn to grasp from humans
Teaching robots to handle objects gracefully and effortlessly like humans has remained a great challenge in robotics. We built new tools to understand the human grasp in detail, in hopes of shaping the next generation of robots and prosthesis.
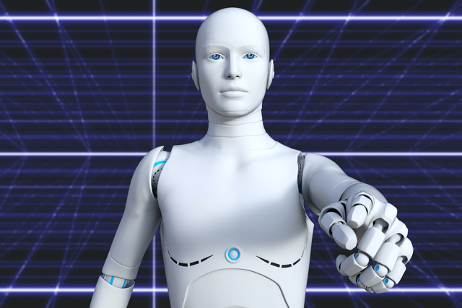
We use our hands every day to hold and move objects of various shapes, sizes, materials and weights. However, grasping an object is a complex process, even though we do it effortlessly without even a second thought. For example, if we want to lift a warm cup of tea, we use a different grip and amount of force than if we want to pick rose petals. While we take our ability to touch and feel things for granted in our daily lives, we do not know how to recreate this in prosthetic implants and modern-day robots. Teaching robots to grasp like humans has been an old dream - and here we studied the human grasp with this in mind.
Today, robots are trained to use visual information to understand the world around them. This is due to the availability of cameras that provide a large amount of data. It has allowed roboticists to train robots using powerful algorithms, called Deep Neural Networks, that mimic how information is processed in the brain. These algorithms help to condense a vast amount of information into meaningful excerpts and instructions. Yet, handling delicate objects with high dexterity has remained challenging.
We grasp and lift things intuitively thanks to many sensors that cover our hands and help us to feel very delicate forces through a process called tactile feedback. Many basic questions about tactile feedback still remain unanswered. To understand how the forces at different points in our hand are used together, we created a sensor array that measures forces at 548 points spread uniformly across the entire human hand. Importantly, we used readily available materials that cost about $10 and simple tools to build it. We attached the sensor array onto a glove that can be worn on the hand, while interacting with 26 common objects, like a ball, a spoon or a stapler. Depending on the object, the force measurements collectively form a tactile map with specific patterns, which allow us to gain insights into the human grasp.
We collected over 135,000 tactile maps over a few hours. Then, we trained a Deep Neural Network to identify objects solely from these tactile maps. This is analogous to how humans identify objects inside a bag (without seeing) by simply touching them. Our algorithm learned to identify an object by looking for consistent patterns, like edges and sharp points, or the use of specific fingers. In other experiments, our algorithm was able to predict the weights of objects or differentiate between various hand poses using tactile data alone.
Results of our study served several purposes. Firstly, these experiments qualify our hardware as a scalable, low-cost tactile sensing platform that can be used over long intervals. We shared the designs for our sensor array online - http://humangrasp.io/ - hoping that it will motivate others to use our hardware for further investigations. Secondly, our experiments helped us to understand the key features, such as edges and blobs, that help identify an object while grasping. These resemble well-known visual patterns that humans use to identify objects. While the similarity between the basic patterns used to identify objects by eye and touch has been expected, it was difficult to observe it directly until now. Thirdly, we present the first numerical evaluation of the cooperation between our fingers at a fine resolution. This information can directly guide the design of future prosthetics and robot arms.
Overall, we characterized some of the key signatures of human grasp. We hope that our work attracts others to this important challenge. In the future, one can imagine the sensitization of large surfaces, ranging from next generation car dashboards to full robot exoskeletons. Reliable large-scale tactile sensor networks and algorithms will become increasingly important and will also have real consequences for future prosthetics. Once we endow robots with "human-like" dexterity, there will be transformative implications across many industries.
Original Article:
Sundaram S, Kellnhofer P, Li Y, Zhu J, Torralba A, Matusik W. Learning the signatures of the human grasp using a scalable tactile glove. Nature. 2019;569(7758):698-702.Next read: Mathematical paradoxes unearth the boundaries of AI by Matthew J. Colbrook , Vegard Antun , Anders C. Hansen
Edited by:
Dr. Monika Stankova , Senior Scientific Editor
We thought you might like
More from Maths, Physics & Chemistry
Reversible Anticoagulants: Inspired by Nature, Designed for Safety
Jun 12, 2024 in Maths, Physics & Chemistry | 4 min read by Millicent Dockerill , Nicolas WinssingerDistance-preserving moves always keep a point fixed
May 18, 2024 in Maths, Physics & Chemistry | 4 min read by Shaula FiorelliA resonance triggers chemical reactions between the coldest molecules
Apr 5, 2024 in Maths, Physics & Chemistry | 3 min read by Juliana Park , Wonyl ChoiNatural products might just be our best weapon against antibiotic resistance
Apr 3, 2024 in Maths, Physics & Chemistry | 3.5 min read by Olivier Kirchhoffer , Jahn Nitschke , Jean-Luc WolfenderHeading underground with cold atoms
Dec 1, 2023 in Maths, Physics & Chemistry | 3 min read by Jamie Vovrosh , Sam Hedges , Farzad HayatiEditor's picks
Trending now
Popular topics